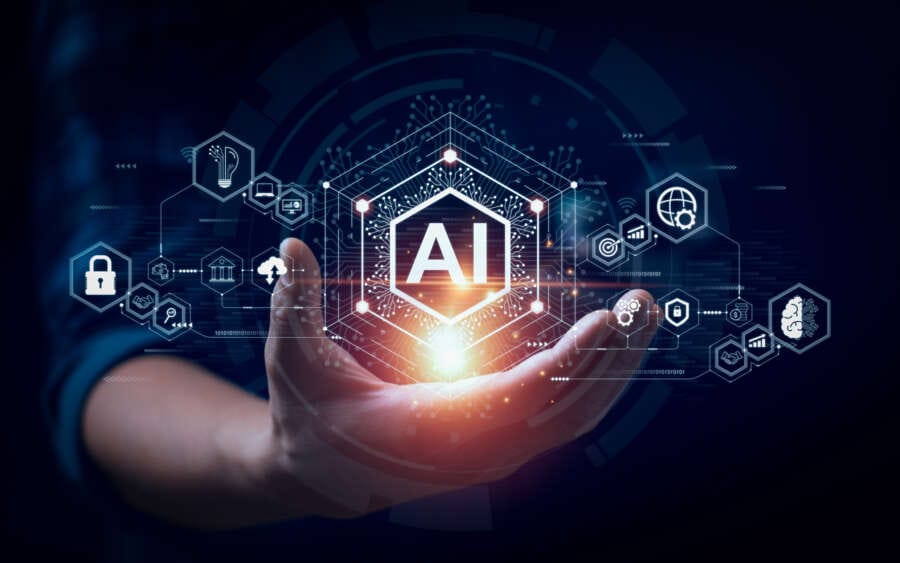
Although the majority of attention rests on Artificial Intelligence applications in the private/for-profit sector, there is a vast scope for AI applications and associated automation to transform the public and not-for-profit sectors. Many continue to rely on legacy manual processes or procedures.
April is a great recent example of one of the most daunting challenges in the public sphere being solved by AI tooling. This AI-enabled tax software allows users to quickly and accurately file their returns without having to search for, read, and interpret IRS regulations. Converting something as complex as the US tax code into a rules engine is an incredible feat – and brings to mind many other opportunities for improvement outside of the ‘for profit’ sector.
Issues with resource deployment
Take, for example, the all-too-frequent scenario of a war-torn or climate-disaster-impacted region that leaves its citizens without access to basic necessities in the near term and without critical infrastructure in the medium term – such as schools, hospitals, and roads. Coming to the assistance of these folks involves predominantly manual processes. Typically, a visual inspection and in-person interviews are conducted to understand the gravity and scope of the situation. These tasks are carried out by various actors, including NGOs, religious organisations, local governments and businesses, each seeking to do their part to provide relief or reconstruction.
Despite the best intentions, a lack of coordination and a centralised dataset indicating the type, volume and location of needs results in redundancies and gaps in effort and resource deployment. The downstream effect of this is that beneficiary needs often fail to be met, such as families without access to a kitchen receiving food aid consisting of dry cereal grain and pasta that they cannot cook or consume. Or donors inadvertently send the wrong types of goods that use supply chain and storage capacity that would be better served otherwise, such as sending winter jackets to the southern hemisphere in December (mid-summer).
Improving aid outcomes
The key to tackling an issue such as this one begins and ends with data. Reliable, timely datasets indicating the location and specific needs of a populace or group provide the key to unlocking optimised aid procurement and delivery, reducing the likelihood of over or under-supply. Similarly, a reliable, timely dataset indicating the location and specific inventory available at stores or aid/relief stations operating in a given region provides visibility to constituents looking to meet their needs and provide support for local businesses. Marrying these two groups is entirely possible with properly structured AI tooling, which enables automated intake of constituent needs and donor or business supplies to facilitate a ‘best match’ within a given region.
Instead of relying on manual inspection and estimates, suppliers and businesses could inform donation requests and inventory purchases with a real-time demand signal from beneficiaries or constituents in their area. In addition to indicating what they need, beneficiaries could be ‘matched’ with the best suppliers or businesses in their area. The end result is a much better alignment of supply and demand, a reduction in waste and an increase in successful outcomes.
Combining AI with existing technology
More positive outcomes are possible thanks to the acceleration of mobile phone penetration globally and the prevalence of 5G. The needs and location of individuals and groups may now be understood at scale via a mobile application or interface. Also critical to the proper tagging and grouping of disparate inventory types at scale is AI, which is increasingly able to assess and categorise individual types of goods and services into manageable groups and used to inform disaster response and demand planning. For example, instead of ‘access to public bus’ ‘access to a taxi’ ‘access to a bike’ answers could be grouped simply as “transportation”.
A similar AI-enabled interface would reduce the friction between local buyers and local ‘bricks and mortar’ sellers, allowing each group to discover what is available around them, which is especially relevant when many stores are closed or permanently damaged and to advertise which stores are open for business.
Using a data-driven demand signal to better match demand and supply from crisis to reconstruction stands to significantly improve overall outcomes in the aid sector, enabling NGOs and providers to “do more with less” and resulting in better specific and long-term outcomes for beneficiaries and constituents.
Lacey Hunter Bio
Lacey Hunter is the CEO and Co-Founder of TechAid, a venture envisioned at the World Economic Forum (2022) that enables a data-driven approach to humanitarian aid and infrastructure to facilitate economic resilience. She believes fundamental human rights should not be dictated by the arbitrary nature of one’s birthplace or gender. Lacey is fascinated with the incredible potential of blockchain and AI to improve outcomes and transparency, reduce inefficiency and waste, and to better serve the needs of the global population.